Did you know 80% to 90% of A/B tests do not produce any statistically significant result? Only 1 out of 8 A/B tests show a significant difference.
Done incorrectly, some marketers have begun to question the value of A/B testing—their A/B test reports an uplift of 20%. Yet, the increase reported by the AB testing software never seems to translate into improvements or profits.
The reason? “Most winning A/B test results are illusory.” (Source: Qubit)
Furthermore, most arguments that call for running A/A testing consider it a sanity check before you run an A/B test.
In this post, we’ll examine arguments around that (for and against it) and suggest other ways to look at A/A tests and why we run them regularly on our CRO projects.
Let’s dive right in!
What is an AA test?
An A/A test is essentially an A/B test where both variations are identical. Instead of comparing different versions of a webpage or marketing material to see which performs better, an A/A test compares two identical variations against each other.
In theory, AA tests are designed to help marketers examine the reliability of the A/B testing tool used to run them, aiming to find “no difference between the control and variant.”
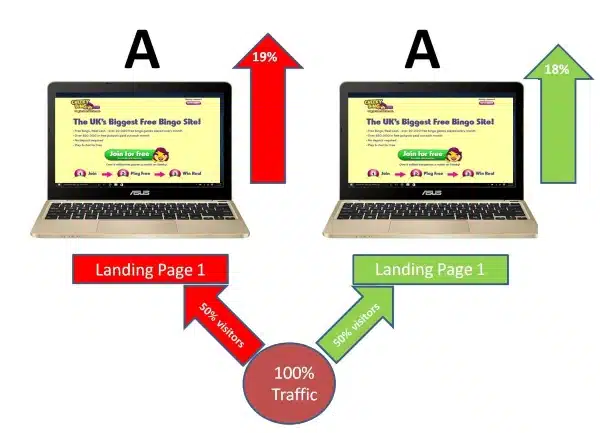
Now, since you’re running the original page against itself (or multiple versions of itself), it will be logical that visitors will react the same way to all the different test recipes. Thus, the A/B testing software will not be able to declare a winner.
The theory is that the difference in conversion rates between variations will not reach statistically significant results.
Other versions of AA tests include running an AABB test. In this case, you will have the control, an identical variations of the control, a challenger, and an identical copy of the challenger.
Then, you will run your A/B test as you usually do with an original and a challenger, but you will also add two sanity check versions to measure the accuracy of the testing software on the results.
How does AA testing work?
Running an AA test is much like running AB tests, except in this case, the two groups of users randomly chosen for each variation are given the same experience.
Here’s a quick breakdown of how AA testing works:
- Create two identical versions: Duplicate your existing webpage, email, or other marketing material exactly.
- Divide traffic: Split your test traffic equally between the two identical versions.
- Monitor results: Track key performance indicators (KPIs) like click-through rates, baseline conversion rate, or revenue generated.
If your A/B testing tool is working correctly, you should see no statistically significant difference between the two identical versions. Any significant difference indicates a potential issue with the tool, your experiment setup, or data quality.
Note: You will also want to integrate your AB testing tool with your analytics to compare conversions and revenue reported by the testing tool to those reported by analytics—they should correlate.
Purpose of AA Testing
Whether to conduct an A/A test or not invites conflicting opinions. Some companies include running an A/A test as part of any engagement, while others consider it a waste of time and resources.
Here are some reasons for companies to run AA tests:
- Validate testing setup: Ensure the testing tool, data collection, and analysis processes are working correctly.
- Identify biases: Detect any inherent biases in the testing methodology or data.
- Establish baseline metrics: Determine expected performance levels for future AB tests.
Arguments against AA Testing
There are three main arguments against running AA testing:
1. Running an AA test is a waste of time and resources that you could use for something that generates better ROI
Craig Sullivan, one of the early CROs, doesn’t recommend A/A testing.
Not because he thinks it is wrong, rather,
“My experience tells me there are better ways to use your time when testing. The volume of tests you start is important, but even more so is how many you *finish* every month and how many from those that you *learn* something useful from. Running A/A tests can eat into ‘real’ testing time.”
The issue is not philosophical for Craig but one that revolves around practicality. This makes total sense in an industry focused on delivering the most value for clients.
While you could technically run an A/A test in parallel with an A/B test, doing so would make the process more statistically complex.
It will take longer for the test to complete, and you’ll have to discard your A/B test results if the A/A test shows that your tools aren’t properly calibrated.
2. Declaring a winner in an A/A test does not tell you a lot
Confidence is Inherent in any type of split or multivariate testing. The fact that an A/B testing engine declares a winner with 99% confidence does not mean that you are certain that you found a true winner.
A statistical significance of 95% means that there is a 1 in 20 chance that the results you’re seeing in your test are due to random chance.
As a matter of fact,
“After running thousands of A/B tests and hundreds of A/A tests, I expected to see different testing platforms regularly declare a winner in an A/A test. I have seen this in Test & Target, Google Website Optimizer (while it lasted), Optimizely, and VWO.”
3. A/A tests require a large sample size to conclude
The final argument against running A/A tests is that they require a large sample size to prove that there is no significant bias.
Here’s a vivid example Qubit shared in their phenomenal white paper titled “Most Winning A/B Test Results Are Illusory:”
Imagine you are trying to determine whether there is a difference between men’s and women’s heights.
If you measured only a single man and a single woman, you would risk not detecting the fact that men are taller than women.
Why is this? Because random fluctuations mean you might choose an especially tall woman or an especially short man just by chance.
However, if you measure 10000 people, the average for men and women will eventually stabilize, and you will detect the difference between them. That’s because statistical power increases with the size of your sample.
The Unexpected Benefits of A/A Testing in CRO
At Invesp, we run A/A tests as part of any CRO services. We typically run these tests at the start of the project and then every 4 to 6 months for the first 1-2 weeks as we gather different data on the website and its customers.
Our rationale:
1. We want to benchmark the performance of different pages or funnels on the website
How many visitors or conversions come to the homepage, cart page, product page, etc?
When we do that, we are not worried about whether we are going to find a winner; we are looking for general trends for a particular page.
These tests help us understand questions such as: What is the macro conversion rate for the home page?
How does that conversion rate break down between different visitor segments? How does that conversion rate break down between different device segments?
A/A tests provide us with a baseline that we can examine when preparing new tests for any part of the website.
We can get the same data from the analytics platforms on the website. Yes, and No!
Since our A/B testing tool is mainly used to declare a winner (while still sending data to Google Analytics or doing external calculations), we still want to see the website metrics when using it.
2. We decide on a minimum sample size and expected time to run a test
Determining the required sample size is very important for an A/B test
If the sample size is too small, little information can be obtained from the test to draw meaningful conclusions.
On the other hand, if it is too large, the information obtained through the tests will be beyond that needed, wasting time and money.
When we conduct an A/A test for different areas of the funnel, we look closely at the number of visitors the A/B testing platform is capturing, the number of conversions, conversion rates, etc.
This data helps us determine the minimum sample size required to run an A/B test on a particular website funnel and how long we need to run our regular A/B tests.
3. We want to get a general sense of how long it takes to deploy the simplest, straightforward A/B test on the website
You have to agree that an A/A test is the easiest and fastest test you can deploy on a website. It is amazing how many technical challenges appear when you run a simple A/A test. This is always the case if the client is just starting with a CRO project.
They have never deployed a test on their website. The more complicated the technical architecture for the client website, the more AA tests will be helpful in identifying possible technical issues before we launch the actual program.
Scripts are not installed correctly, GTM needs to be configured to capture additional data, issues around third-party conversions, and the list goes on.
4. Never trust the machine: check the accuracy of the A/B testing tool
Before running A/B tests, it’s important to make sure your tools are configured correctly and working the way they should.
Running these tests helps us check the accuracy of the A/B testing tools we’re using.
What more?
Companies about to purchase an A/B testing tool or want to switch to a new testing software may run an A/A test to ensure the new software works fine and to see if it has been set up properly.
I recall one project where all tests run by the client on a particular testing platform ended up with a loss. All 170 tests. Mind you, I am used to running tests that generate any improvement. But running 170 tests with no result is unusual.
When we switched the client to another platform and re-ran some of the ten most promising tests, 6 of them resulted in the winner with 99% confidence.
Chad Sanderson, co-founder and CEO of Gable.ai, had great insights into this:
It isn’t wise to downplay the danger of system errors. Most A/B testing solutions use slightly different algorithms that may or may not result in major discrepancies the harder the program is pushed (Think 10 – 20 – 30 variants). This might seem like an outlier issue, but it also might indicate a deeper underlying problem with either A.) the math B.) the randomization mechanism, or C.) the browser cookie. Tools break (quite often, actually), and putting blind trust in any other product is asking for trouble.
We have to admit that the reliability of your AB testing software is a scary thought. If you are using that AB testing software to determine the winner of your tests, and then you question the reliability of the software, you are effectively questioning your whole program:
Chad Sanderson adds:
If a program doesn’t generate an overwhelming amount of type I errors (95% confidence), it doesn’t mean it still can’t be flawed. Thanks to the statistical mechanisms behind A/A tests (P Values are distributed uniformly under the null hypothesis), we can analyze test data the same way we’d determine whether or not a coin is fair or weighted: by examining the likelihood of observing a certain set of outcomes.
For example, after flipping a fair coin 10 times, we could expect to see 10 heads in a row only once out of 1024 attempts (50/50 chance per flip). In the same way, if we run a 10 variant A/A test and see all 10 values have a p-value over .5, the probability of this happening would be the same (50/50 chance per test). Without going too deep into Bayesian statistics, the next step would be to ask yourself if it’s more likely that you observed a rare result on your first attempt or that something is wrong with the tool.
If you’re a CRO, let me suggest a new idea. Do not rely on your A/B testing software to declare winners for the next month. Send your testing data to Google Analytics, pull the numbers for each variation from analytics, and do the analysis yourself!
Considerations when conducting A/A testing
1. What should you do if your A/A test shows a winner?
When running an A/A test, it’s important to remember that finding a difference in conversion rate between two identical versions is always possible.
This doesn’t necessarily mean the A/B testing platform is inefficient or poor, as there is always an element of randomness in testing.
Now, what should you do if you find a winner?
Let me give you an example posted by Chad Sanderson using Adobe Target, running 10 Variants, all default vs default.
The period being looked at here was 3 weeks (note the number of orders on the left and confidence to the right) on a Desktop – New Visitors – Cart segment. Running these through a one-tailed statistical calculator, even with a strict family error correction, still yields 7 significant results.
If you used a Bayesian interpretation, it would be 10/10. All variants had more than enough power. Yikes.
2. If you perform A/A tests and the original wins regularly,
There is a good chance that challengers are losing due to performance issues (it takes a little bit to load a variation, and that delay works in favor of the original).
Therefore, evaluate your testing platform. We have seen this happen more with some testing platforms than others.
3. If you perform A/A tests regularly, and one of the variation/original wins:
It is a fact of life! In my experience, testing engines will declare a winner in 50% to 70% of AA tests (95% to 99% significance).
But then, look at the kind of uplifters and downlighters you’re seeing: If one of the variations wins with 3-4% regularly, that could be a signal that you should aim for uplifts higher than that when running your tests.
Chad Sanderson adds a great point:
Another great use of A/A Tests is as physical evidence for or against testing a page or element. Let’s say you’ve run an A/A test and, after one month, have observed a difference between means that is still large, perhaps over 20%. While you could determine this just as easily from a sample size calculation, presenting numbers without context to stakeholders who REALLY want to run tests on that page may not get the job done.
It’s far more effective to show someone the actual numbers. If they see that the difference between two variations of the same element is drastic even after a month, it’s far easier to understand why observing test results on such a page would mean only a result far greater than what was observed would be possible.
In this case, you’ll have the control, the challenger, and an identical copy of the control.
Final Thoughts:
Whether you believe in A/A testing or not, you should always run a winner from an A/B test against the original in a head-to-head test to validate wins.
Here’s an example:
You run an A/B test with 4 challengers against original V2 wins with a 5% uplift, then create a new test with the original against V2 in a head-to-head test.
Done correctly, A/A testing can help prepare you for a successful AB testing program by providing data benchmarks on different areas of the website and checking for any discrepancy in your data, let’s say, between the number of visitors you see in your testing tool and the web analytics tool.
What’s your perception of A/A tests? Are you running them on your website? I’d love to hear your thoughts, so let me know in the comment section below!
Additional Resources
- What Is Conversion Rate Optimization?
- What is AB Testing?
- What Is Multivariate Testing?
- What Are The Features Of A Good AB Testing Tool?